President Biden’s recent Executive Order on AI responds to the promise and peril surrounding generative AI by creating safeguards to ensure privacy, justice, and security which must also address the climate risks of AI and help limit the growth of emissions and climate disinformation from AI technologies.
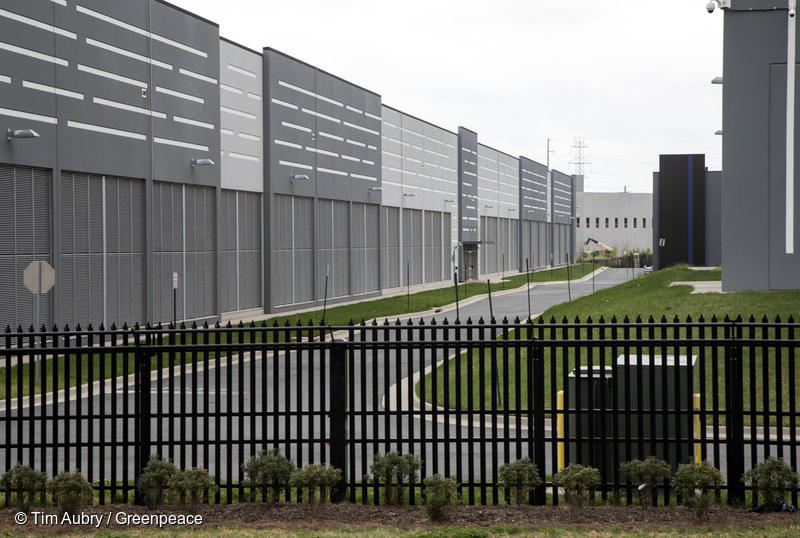
Tech companies are racing to develop artificial intelligence (AI) technologies that could radically change how we work and communicate. The release of powerful generative AI products like Open AI’s ChatGPT has sparked excitement about the many potential useful applications but has also triggered alarms about the threat to privacy, security, and democracy, and highlighted the lack of oversight. Six months after telling top executives “what you’re doing has enormous potential and enormous danger,” President Joe Biden began laying the groundwork for putting safeguards around AI by issuing an Executive Order that requires federal agencies create standards and guidance to ensure privacy and security, and companies to report information about risks.
Policies to address the risks and ethical challenges raised by generative AI, even arising from the industry itself, must also address the environmental and climate risks. Currently data centers represent 1-1.5% of global electricity use, but that could grow significantly with rising demand for computational power driven by the boom in AI and digital mining for cryptocurrencies like Bitcoin. Biden’s Executive Order should be the first step in a process that holds tech companies accountable and protects the public and environment by requiring stronger disclosure standards and other measures that limit energy use, associated carbon emissions, and other environmental impacts from AI, including large water use and the consumption of metals and minerals like lithium.
Back in 2017, referring to its revolutionary potential to transform virtually all aspects of our economy and lives, computer scientist Andrew Ng described AI as “the new electricity.” Although he wasn’t referring to energy use from rapid adoption of AI, researchers are now finding that AI can be very energy and carbon intensive. Generative AI tools that use large language models (LLMs), such as ChatGPT or Microsoft’s AI-enhanced Bing, require lots of computing power and thus, electricity. According to industry insiders, a data center running AI tasks could consume three to five times more energy than traditional data centers. While researchers and industry analysts have identified the potential energy bomb from AI, there are still many questions and unknowns. Better data and greater transparency from secretive companies is needed to accurately measure AI’s electricity use and evaluate the climate and environmental risks.
The energy consumption from generative AI occurs during two phases – “training” or creating an AI model and then “inference” or the use of an AI model. Training a LLM requires feeding it huge datasets to process, which can take several weeks to several months and requires tens of thousands of advanced micro-chips called graphics processing units (GPUs) that use large amounts of electricity. Researchers found that training just one model, GPT-3, took 1,287 gigawatt hours which is as much electricity as 120 U.S. homes use in a year, and generated 552 tons of carbon which is equivalent to emissions from 110 gas-powered cars on U.S. roads in a year. Another team estimated that training GPT-3 consumed about 185,000 gallons of water — equivalent to what about 2,200 average Americans consume in their homes over a year. That is just one AI model, but many other companies and researchers are developing their own AI systems that are even more complex, relying on more computing power.
Yet, training is only the first step. Using generative AI models also requires electricity, potentially much more than what is used in the training phase. Google analysts estimate that training was only about 40% of the energy used by their generative AI while the other 60% came from running queries, although this will change depending on the popularity of the AI model and its complexity. The energy to run the millions and billions of queries that popular programs like Chat GPT receive adds up quickly. An academic paper estimated that OpenAI uses 564 MWh per day to support the use of Chat GPT-3 which would take only three days to surpass the estimated 1,287 MWh used in training GPT-3.
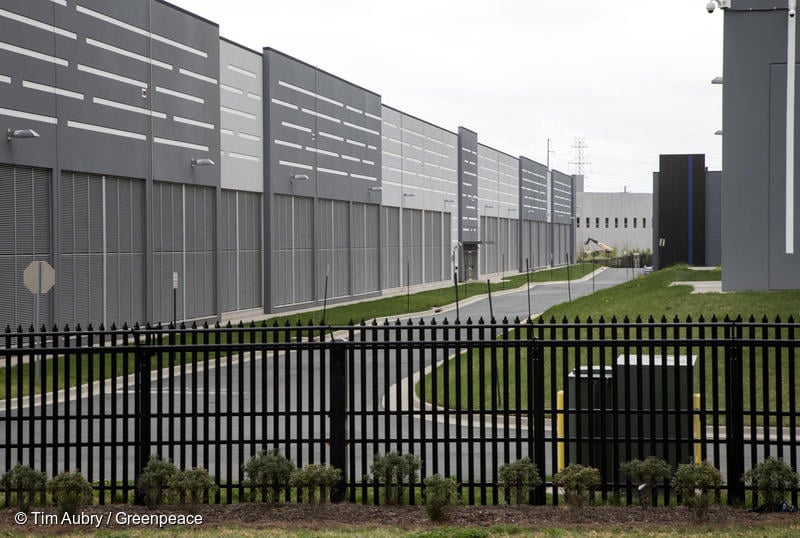
An Amazon World Services Data Center in Ashburn, Virginia.
Running search engines with generative AI could increase the electricity needed to power people’s regular Internet use. According to an industry analyst, a single generative AI query could use 4 to 5 times more energy than a regular search engine query. Others found that average energy consumption per search could be 6.9–8.9 Wh, compared to 0.3 Wh for a standard Google search. Research and consulting firm SemiAnalysis estimates that integrating generative AI into search engines like Google, which runs up to nine billion searches per day, could lead to a daily electricity consumption of 80 GWh and an annual consumption of 29.2 TWh, equivalent to what about 2.7 million average U.S. households use in a year. A worst-case scenario in which Google’s search engine ran entirely on AI could consume as much electricity as Ireland.
Researchers have attempted to estimate the current and future energy, carbon, and water footprint of AI. A scenario in an academic paper finds AI servers could use between 85 and 134 terawatt hours annually by 2027 which is comparable to what Argentina or Sweden uses annually, and about 0.5 percent of current global electricity consumption. In many locations, much of this electricity creates carbon emissions since it’s powered by dirty fossil fuels. A team of academics predict that global AI water demand could reach 4.2–6.6 billion cubic meters of water by 2027, which is half of the United Kingdom.
Many factors will influence how much energy and water is used by AI and the rate at which it grows. The electricity required depends on the design of the model, the type of processors used, and the data center facility. The carbon emissions also depend on the fuel sources powering data centers and where they are located. Researchers from Google and University of California-Berkeley found that using more efficient model architecture, equipment, and greener data centers could reduce the carbon footprint of an AI model by 100 to 1,000 times. When and where AI models are trained also impacts the water intensity and smart decisions can reduce water use.
One immediate conclusion from these and other studies is that, in order for AI systems to be designed and developed in ways that minimize environmental impacts, more transparency and reporting of energy consumption and emissions will be needed. Currently, such estimates are hampered by a lack of quality data and opaque company operations. AI has potential to help address the climate crisis with applications in areas like smart grids and renewable energy technologies, but it could also drive up emissions if there is no oversight and regulatory guidance.
President Biden has described climate change as “literally an existential threat to our nation and to the world,” a full-spectrum threat to the nation’s national security, infrastructure, economy, public health and workplace safety. It’s time to square the circle: As the administration starts developing and implementing strategies to prevent other serious potential AI-related threats including fraud, racial discrimination, the invasion of privacy and deep fakes and other threats to the integrity of our elections, it must also develop strategies to prevent AI from creating a perfect storm of climate misinformation. These should include setting rigorous disclosure standards for energy consumption and associated emissions (as some AI developers have suggested) along with setting energy efficiency and renewable generation procurement standards, and exploring additional ways we can prevent the rapid deployment of AI from driving up emissions and hurtling us towards a perpetual climate catastrophe that no language learning model could ever find the right words to describe.